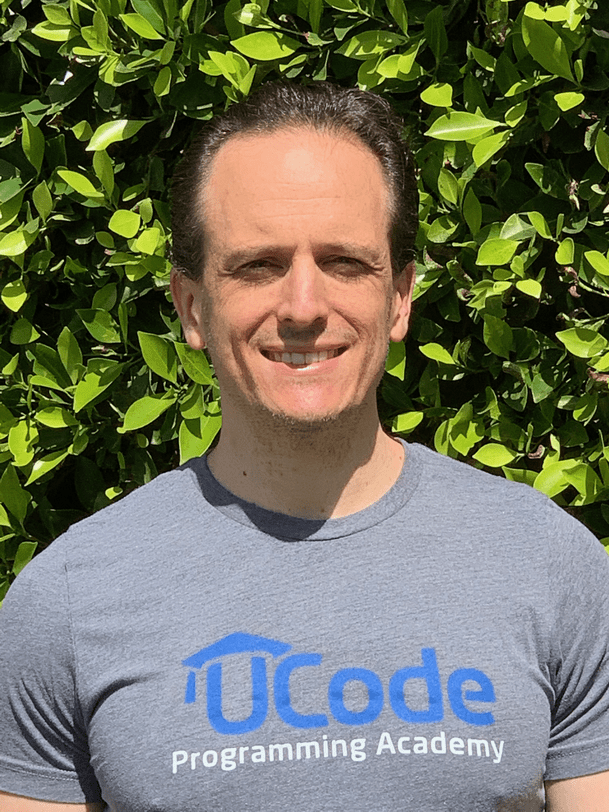
- Scott Allen Mueller
- scott@cs.ucla.edu
- PhD candidate
- Computer Science dept
- UCLA
About Me
I'm a perpetual student of Causality and AI. After a career as a tech entrepreneur, I returned to academia to pursue a PhD with the aim of contributing to the development of AGI. My journey took a pivotal turn when I read The Book of Why by Judea Pearl, which caused an obsession with causal inference. I was extraordinarily fortunate to have Dr. Pearl become my PhD advisor, and my admiration and respect for this living legend continue to grow unbounded.
My research focuses on personalized decision making with counterfactual reasoning. We are born with innate cause and effect instincts, experimenting with the world causally even as infants. However, we seldom develop counterfactual reasoning skills, which can severely impact our decision-making abilities. I believe that incorporating this capability into machine learning algorithms will allow us to achieve superhuman-level reasoning.
Previously, I founded UCode, an initiative to teach computer science to kids and teenagers. After seven years of improving my understanding of teaching advanced concepts to young students, I sought to comprehend intelligence at a fundamental level. I believe that causality is essential to intelligence, reasoning, decision-making, and ultimately, the realization of AGI.
Papers
-
March 2025
Personalized and Situation-Specific Decision Making
ProQuestPh.D. Dissertation
Personalized and situation-specific decision making is a framework that optimizes both population-based and individual-based utilities by leveraging causal knowledge and counterfactual reasoning. This dissertation addresses the shortfalls of traditional decision strategies, which often overlook crucial individual heterogeneity. It develops novel methods of estimating how specific people or cases would respond under alternative actions or treatments, then uses those estimates to optimize decisions. By combining evidence from both experiments and observational studies, the approach side-steps the fundamental limitation of observing only one outcome per individual. As a result, an individual's probability of benefiting from, or being harmed by, an intervention can be estimated more precisely than previously possible.
The proposed methods also incorporate domain knowledge through causal models, and culminate in a practical way to handle non-binary ordinal outcomes, substantially expanding the usefulness of counterfactual reasoning. This framework is validated on real-world data from Tennessee's STAR project. Population-level data is distilled into individual-level probabilities, which in turn sharpen both broad policy decisions and personalized choices. I show how the benefits of this framework extend across virtually every industry and discipline. -
February 2024
Causal AI Framework for Unit Selection in Optimizing Electric Vehicle Procurement
Presented at: AAAI 2024 Workshop on Sustainable AI
Electric vehicles (EVs) are generally considered more environmentally sustainable than internal combustion engine vehicles (ICEVs). Government and policy makers may want to incentivize multi-vehicle households who, if they purchase a new EV, would use their EV to replace a large portion of their ICEV mileage. Therefore, it is important to analyze how EV procurement affects annual EV mileage for different households. Given that many relevant data, especially experimental data, are often unavailable in the real world, we need causal analysis tools to answer this question. Additionally, our aim is to compare the expected EV mileage of different combinations of vehicles a household owns. Observing multiple combinations in an individual household is impossible since only one combination can exist, making causal inference challenging. In this paper, we construct a causal AI framework utilizing counterfactual reasoning methods to address this issue.
-
August 2023
Perspective on ‘Harm’ in Personalized Medicine – An Alternative Perspective
American Journal of EpidemiologyThis commentary examines an article by Sarvet and Stensrud (SS), in which they discuss the concept of ‘harm’ and its application in medical practice. SS advocate for an intervention-based interpretation of harm, downplaying its counterfactual interpretation. We take issue with this stance. We show that the counterfactual approach is vital for effective decision-making policies and that neglecting it might lead to flawed decisions. In response to SS’s contention that “when the outcome is death and a counterfactual approach is used … more people will die,” we demonstrate how counterfactual reasoning can actually prevent deaths. Additionally, we highlight the advantages of counterfactual thinking in the fields of medical malpractice, legal reasoning, and general diagnoses. Relying solely on intervention-based analyses limits our ability to accurately represent reality and hinders productive discussions about evidence, assumptions, and consensus building.
-
August 2023
Monotonicity: Detection, Refutation, and Ramification
Presented at: 2023 RAND Center for Causal Inference (CCI) Symposium
The assumption of monotonicity, namely that outputs cannot decrease when inputs increase, is critical for many reasoning tasks, including unit selection, A/B testing, and quasi-experimental econometrics. It is also vital for identifying Probabilities of Causation, which, in turn, enable the estimation of individual-level behavior. This paper demonstrates how monotonicity can be detected (or refuted) using observational, experimental, or combined data. Using such data, we pinpoint regions where monotonicity is definitively violated, where it unequivocally holds, and where its status remains undetermined. We further explore the consequences of monotonicity violations, especially when a maximum percentage of possible violation is specified. Finally, we illustrate applications for personalized decision-making.
-
March 2023
Personalized Decision Making -- A Conceptual Introduction
Journal of Causal Inference 2023 (Volume 11 Issue 1)Personalized decision making targets the behavior of a specific individual, while population-based decision making concerns a subpopulation resembling that individual. This article clarifies the distinction between the two and explains why the former leads to more informed decisions. We further show that by combining experimental and observational studies, we can obtain valuable information about individual behavior and, consequently, improve decisions over those obtained from experimental studies alone. In particular, we show examples where such a combination discriminates between individuals who can benefit from a treatment and those who cannot – information that would not be revealed by experimental studies alone. We outline areas where this method could be of benefit to both policy makers and individuals involved.
Addendum: Personalized Decision Making under Concurrent-Controlled RCT Data
-
January 2023
ε-Identifiability of Causal Quantities
Identifying the effects of causes and causes of effects is vital in virtually every scientific field. Often, however, the needed probabilities may not be fully identifiable from the data sources available. This paper shows how partial identifiability is still possible for several probabilities of causation. We term this ϵ-identifiability and demonstrate its usefulness in cases where the behavior of certain subpopulations can be restricted to within some narrow bounds. In particular, we show how unidentifiable causal effects and counterfactual probabilities can be narrowly bounded when such allowances are made. Often those allowances are easily measured and reasonably assumed. Finally, ϵ-identifiability is applied to the unit selection problem.
-
June 2022
Causal Inference in AI Education: A Primer
Journal of Causal Inference 2022 (Volume 10 Issue 1)The study of causal inference has seen recent momentum in machine learning and artificial intelligence (AI), particularly in the domains of transfer learning, reinforcement learning, automated diagnostics, and explainability (among others). Yet, despite its increasing application to address many of the boundaries in modern AI, causal topics remain absent in most AI curricula. This work seeks to bridge this gap by providing classroom-ready introductions that integrate into traditional topics in AI, suggests intuitive graphical tools for the application to both new and traditional lessons in probabilistic and causal reasoning, and presents avenues for instructors to impress the merit of climbing the “causal hierarchy” to address problems at the levels of associational, interventional, and counterfactual inference. Finally, this study shares anecdotal instructor experiences, successes, and challenges integrating these lessons at multiple levels of education.
-
June 2021
Estimating Individualized Causes of Effects by Leveraging Population Data
Master's Thesis
Most analyses in the past three decades concerned estimating effects of causes (EoC). Less emphasis has been placed on identifying causes of effects (CoE), despite their critical importance in science, medicine, public policy, legal reasoning, AI, and epidemiology. For example, personalized medicine concerns the probability of a drug being the cause of survival: resulting in a favorable outcome if taken and unfavorable if avoided. One reason for this imbalance is that tools for estimating the probability of causation from data require counterfactual logic. Bounds on these probabilities are often too loose to be informative and the assumptions necessary for point estimates are often too strong to be defensible. The objective of this thesis is to develop and test techniques for achieving narrower bounds on the probabilities of causation, with minimal assumptions. These more accurate estimates are achieved by incorporating a causal model and covariate data.
-
May 2022
Causes of effects: Learning individual responses from population data
Proceedings of the Thirty-First International Joint Conference on Artificial Intelligence Main TrackPresented at: IJCAI-ECAI 2022, the 31st International Joint Conference on Artificial Intelligence
The problem of individualization is crucial in almost every feld of science. Identifying causes of specifc observed events is likewise essential for accurate decision making as well as explanation. However, such tasks invoke counterfactual relationships, and are therefore indeterminable from population data. For example, the probability of benefting from a treatment concerns an individual having a favorable outcome if treated and an unfavorable outcome if untreated; it cannot be estimated from experimental data, even when conditioned on fine-grained features, because we cannot test both possibilities for an individual. Tian and Pearl provided bounds on this and other probabilities of causation using a combination of experimental and observational data. Those bounds, though tight, can be narrowed signifcantly when structural information is available in the form of a causal model. This added information may provide the power to solve central problems, such as explainable AI, legal responsibility, and personalized medicine, all of which demand counterfactual logic. This paper derives, analyzes, and characterizes these new bounds, and illustrates some of their practical applications.
Scott Mueller, Ang Li, Judea Pearl
Revision: January 2024
Teaching
- Learning and Reasoning with Bayesian Networks
- Teaching Assistant
- UCLA CS 262A, Winter 2024
- Professor Adnan Darwiche
- Graduate course
- Learning and Reasoning with Bayesian Networks
- Teaching Assistant
- UCLA CS 262A, Winter 2023
- Professor Adnan Darwiche
- Graduate course
- Learning and Reasoning with Bayesian Networks
- Teaching Assistant
- UCLA CS 262A, Winter 2022
- Professor Adnan Darwiche
- Graduate course
- Automated Reasoning: Theory and Applications
- Teaching Assistant
- UCLA CS 264A, Fall 2021
- Professor Adnan Darwiche
- Graduate course
Articles
- Personalized Decision Making under Concurrent-Controlled RCT Data (March 2023)
- Personalized Decision Making (April 2021)
- Which Patients are in Greater Need: A counterfactual analysis with reflections on COVID-19 (April 2020)
- Fréchet Inequalities – Visualization, Applications, and History (November 2019)
Talks/Interviews
- Causal AI & Individual Treatment Effects (episode 20 of Causal Bandits Podcast)
Labs
- Practical Causal Inference (PCI) Lab
- Web Developer and Co-Manager
- Directors: Chad Hazlett and Onyebuchi Arah
- Cognitive Systems Laboratory
- Graduate Student Researcher
- Director: Judea Pearl
CV
PDF or go to my LinkedIn profile